The Insights Leader's Guide to Generative AI
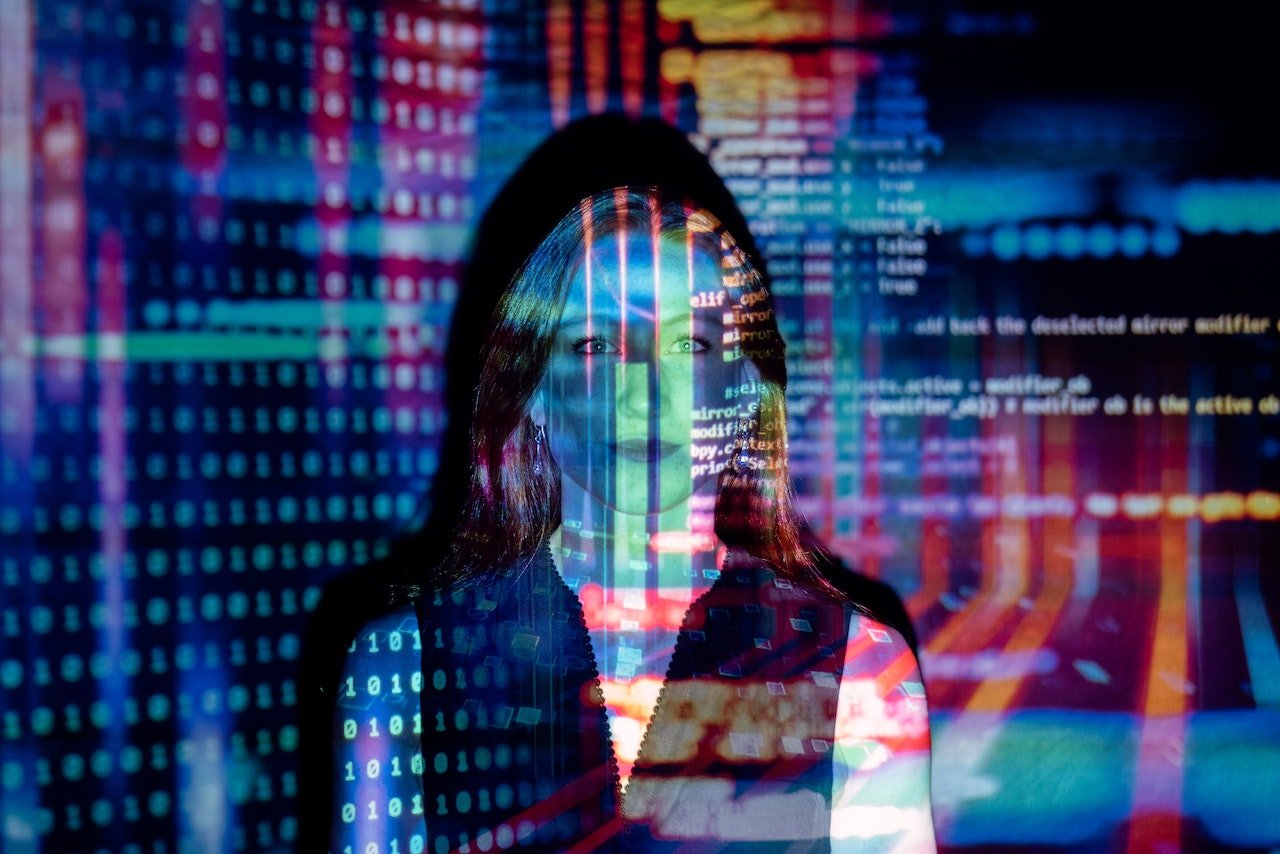
Author's note: The capabilities and implications of Generative AI are constantly evolving, so we'll be updating this guide on a regular basis.
“With great power comes great responsibility.”
You don’t have to be a Marvel buff to recognize that quote, popularized by the Spider-Man franchise.
And while the sentiment was originally in reference to superhuman speed, strength, agility, and resilience, it’s a helpful one to keep in mind when making sense of the rise of generative AI.
While the technology itself isn’t new, the launch of ChatGPT put that technology into the hands of 100 million people in the span of just 2 months, something that for many felt like gaining a superpower. The underlying technology has already become more powerful, as seen just the other week with the release of GPT-4, and the number of possible use cases continues to increase exponentially as seen with the recent release of OpenAI’s new plug-in system.
But like all superpowers, what matters is what you use them for. Generative AI is no different. There is the potential for great, for good, and for evil.
Organizations now stand at a critical juncture to decide how they will use this technology. Ultimately, it’s about taking a balanced perspective – seeing the possibilities but also seeing the risks, and approaching both with an open mind.
In this article, we’ll explore both the possibilities and risks of generative AI for insights teams, and equip you with the knowledge you need to make the right decisions that will move your team forward.
Quick recap: Generative AI in 1 minute or less
If you’ve been keeping up-to-date on the latest with generative AI, GPT-3&4, and LLMs, feel free to skip this part and go to the next section.
Before we dig into the “so what’s?”, let’s quickly revisit the “what’s”.
What is generative AI?
Generative AI refers to deep-learning algorithms that are able to produce new content based on data they’ve been trained on and a prompt. While traditional AI systems are made to recognize patterns and make predictions, generative AI can create new content like text, code, audio, and images.
What are large language models (LLMs)?
A large language model is a type of machine learning model that can perform a variety of natural language processing tasks, like generating/classifying text, answering questions, and translating text. Large language models are the technology behind generative AI. OpenAI has GPT-4, Google has LaMDA, and Meta (Facebook) recently announced LLaMA.
And ChatGPT?
You probably know this one, but just in case: It’s an AI-powered chatbot that uses OpenAI’s GPT-3 model (though GPT-4 was released on March 14, 2023, and is available to ChatGPT plus users).
What it means for insights work
The insights industry is no stranger to change – the tools and methodologies available to insights professionals have evolved rapidly over the past few decades.
At this stage, the extent and speed of the changes that increasingly accessible generative AI will bring are something we can only speculate on. But there are certain foundations to have in place that will help you figure out how to respond quickly as more information becomes available.
Ultimately, it all comes back to asking the right questions, a skill which insights professionals are experts in.
What are the opportunities?
Currently, the primary opportunity offered by generative AI is enhanced productivity. It can drastically speed up the processes of generating ideas, information, and written texts, like the first drafts of emails, reports, or articles. By creating efficiency in these areas, it allows for more time to be spent on tasks that are more important and require human expertise.
Faster time to insight
For insights work specifically, one area we see a lot of potential in is summarization of information. For example, the Stravito platform has already been using generative AI to create auto-summaries of individual reports, removing the need to manually write an original description for each report.
We also see potential to develop this use case further with the ability to summarize large volumes of information to answer business questions quickly, in an easy to consume format, that could cut time-to-insight significantly. For example, this could look like typing a question into the search bar and getting a succinct answer based on your internal knowledge that links to additional sources.
For insights managers, this would mean being able to answer simple questions more quickly, and it could also help take care of a lot of the ground work when digging into more complex problems.
[Learn more about how Stravito uses Generative AI for faster time to insight]
Insights democratization through better self-service
If generative AI technology like this was made available to business stakeholders, it could make it easier for them to access insights without needing to directly involve an insights manager each time. By removing barriers to access, generative AI could help to support organizations who are on an insights democratization journey.
It could also help to alleviate common concerns associated with insights democratization, like business stakeholders asking the wrong questions. In this use case, generative AI could be used to help business stakeholders without research backgrounds to ask better questions by prompting them with relevant questions related to their search query.
This could also extend to even more relevant recommendations of content, and the ability to use questions instead of keywords to get more precise search results.
[Learn how Stravito uses generative AI to help you ask better questions]
Tailored communication to internal and external audiences
Another opportunity that comes with generative AI is the ability to tailor communication to both internal and external audiences. For example, Christopher Graves, founder and president of the Ogilvy Center for Behavioral Science has successfully used generative AI to craft messaging that resonates better with “the Hidden Who”.
In an insights context, there are several potential applications.
It could help make knowledge sharing more impactful by making it easier to personalize insights communications to various business stakeholders throughout the organization.
It could also be used to tailor briefs to research agencies as a way to streamline the research process and minimize the back and forth involved.
What are the risks?
As you’re likely aware, there are also many risks associated with generative AI in its current state. In this section, we’ll explore examples of these risks, like prompt dependency, trust, transparency, and security.
Prompt dependency
One fundamental risk is the prompt dependency. Generative AI is statistical, not analytical, so it works by predicting the most likely piece of information to say next. If you give it the wrong prompt, you’re still likely to get a highly convincing answer. For example, we asked ChatGPT to tell us about the founders of Stravito, and this is what we got:
There is truth in this answer (like the fact that Stravito provides knowledge management for enterprises, and two of Stravito’s founders are Thor Olof Philogène and Anders Örbom).
However, their roles and experience are completely incorrect. For example, Thor has never been the CTO of Stravito, nor has he worked at Jayway or TAT. Anders was never a management consultant, nor has he been a partner at QVARTZ. On top of that, the other founders of Stravito, Andreas Lee and Sarah Lee, were omitted entirely, providing the misleading impression that Stravito was only founded by two people.
Of course, the prompt we used was far from perfect – it was open-ended and didn’t give much information. We continued the conversation with ChatGPT and tried to give it a chance to correct itself:
While ChatGPT acknowledged its mistake when told that it was incorrect, this new prompt created a whole host of new inaccuracies.
Because we prompted it that there were 2 more founders, it “wanted” to give us the answer we were looking for. So it invented a person with a reasonable name and background: Anna Forss, Stravito co-founder and Chief Product Officer (there has never been an Anna Forss at Stravito, as Chief Product Officer or otherwise).
The incomplete and leading prompts we used led the model to create a convincing blend of fact and fiction.
Trust
As demonstrated in the example above, the information that generative AI provides isn’t always correct, a tendency otherwise known as hallucination. What becomes even trickier is the way that it can blend correct information with incorrect information. For example, a person who was somewhat familiar with Stravito might see the right names and company description and assume that the entire answer is correct.
In low stakes situations (like this one), this can be amusing. But in situations where million dollar business decisions are being made, the inputs for your decisions need to be trustworthy.
It’s also worth noting that ChatGPT is only trained on information through the end of 2021, which means that it won't take current events and trends into account.
Additionally, many questions surrounding consumer behavior are complex. While a question like “How did millennials living in the US respond to our most recent concept test?” might generate a clear-cut answer, deeper questions about human values or emotions often require a more nuanced perspective. Not all questions have a single right answer, and when aiming to synthesize large sets of research reports, key details could fall between the cracks.
Transparency
Another key risk to pay attention to is a lack of transparency regarding how algorithms are trained. For example, ChatGPT cannot always tell you where it got its answers from, and even when it can, those sources might be impossible to verify or not even actually exist.
And because AI algorithms, generative or otherwise, are trained by humans and existing information, they can be biased. This can lead to answers that are racist, sexist, or otherwise offensive. For organizations looking to challenge biases in their decision making and create a better world for consumers, this would be an instance of generative AI making work less productive. In the best case scenario, you’ve wasted your time. In the worst case scenario, you make the wrong business decision.
Security
Some of the common current use cases for ChatGPT are using it to generate emails, meeting agendas, or reports. But putting in the necessary details to generate those texts may be putting sensitive company information at risk.
In fact, an analysis conducted by security firm Cyberhaven found that of 1.6 million knowledge workers across industries, 5.6% had tried ChatGPT at least once at work, and 2.3% had put confidential company data into ChatGPT. Companies like JP Morgan, Verizon, Accenture and Amazon have banned staff from using ChatGPT at work over security concerns. And just a few days ago, Italy became the first Western country to ban ChatGPT while investigating privacy concerns, drawing attention from privacy regulators in other European countries.
For insights teams or anyone working with proprietary research and insights, it’s essential to be aware of the risks associated with inputting information into a tool like ChatGPT, and to stay up-to-date on both your organization’s internal data security policies and the policies of providers like OpenAI.
6 questions on generative AI to guide you on your way forward
Generative AI offers both intriguing opportunities and clear risks for businesses, and there is still a lot that is unknown.
As an insights leader, you have the opportunity to show both your team and your broader organization what responsible experimentation looks like. We’ve entered a new era of critical thinking, something that insights professionals are well-practiced in.
The path forward is to ask the right questions, and maintain a healthy dose of skepticism without ignoring the future as it unfolds in front of you. Questions like:
1. How have my team and I been using ChatGPT or other generative AI tools?
The way forward: A good place to start is by seeing the areas where you’re naturally drawn to using these tools. Before you invest in any solution, you want to make sure that generative AI will actually fit into your workflows.
Likewise, it’s a good idea to gauge how open you and your team are to incorporating these technologies. This will help you determine if there need to be more guardrails in place or conversely more encouragement to experiment responsibly.
2. Where are current areas of inefficiency in our intra-team or inter-team workflows?
The way forward: Sketch out the inefficiencies in your workflows, and explore whether it’s something you could automate in whole or in part. These are likely areas where generative AI could offer your team a major productivity boost.
3. What are the most important initiatives currently for our team? For our organization?
The way forward: Chances are you don’t have time for endless experimentation. A good way to focus your exploration is to look at the top priorities for your team and your organization. Focus your efforts where they will have the most impact.
4. What risks do we see as a function and organization with generative AI?
The way forward: Be sure to clearly outline the risks for your function and organization, and don’t hesitate to get advice from relevant experts in tech or security. Once the main risks are defined, you can align on the level of risk you’re willing to tolerate.
5. What opportunities do we see as a function and organization with generative AI?
The way forward: While minding the risks, don’t be afraid to ask the good kind of “What if…?” questions. If you see opportunities, be brave enough to share them. Now is the time to voice them. Likewise, listen to other parts of the organization to see what opportunities they’ve identified and see what you can learn from them.
6. What are we afraid of missing out on as a function and organization with generative AI?
The way forward: You don’t want to be an ostrich with your head buried in the sand, but you should stay mindful of any generative AI FOMO. Make decisions based on what’s best for your organization and your consumers, rather than just copying others.
It’s still our firm belief that the future of insights work will still need to combine human expertise with powerful technology. The most powerful technology in the world will be useless if no one actually wants to use it – this hasn’t changed. But what the most successful constellations will look like remains to be seen.
The focus now should be on responsible experimentation, to find the right problems to solve with the right tools, and not to simply implement technology for the sake of it.
With great power comes great responsibility. As an insight leader, now is your chance to decide how you will use yours.
Learn more about how Stravito is using Generative AI to make insights work better
Related Content
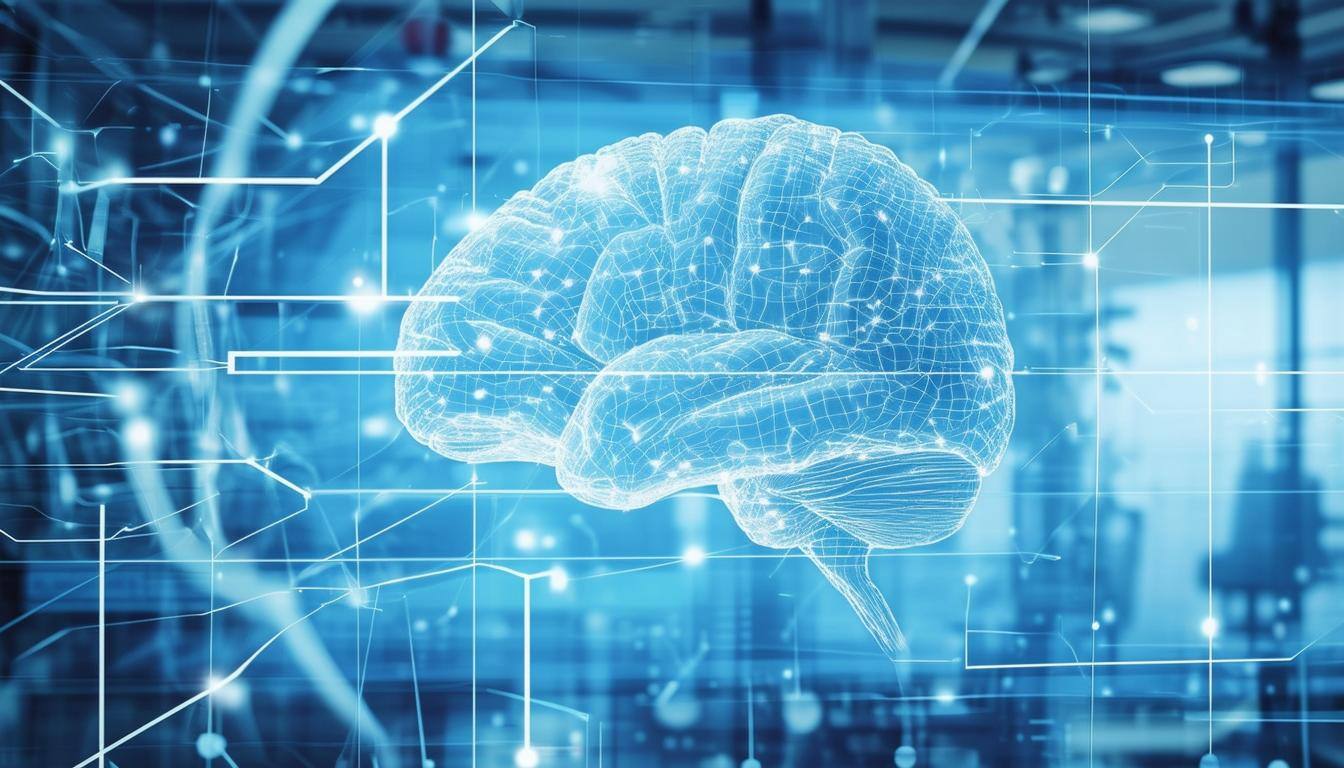
How Roche is Partnering with Stravito to Work Against Insights Inflation
Stravito Oct 31, 2024
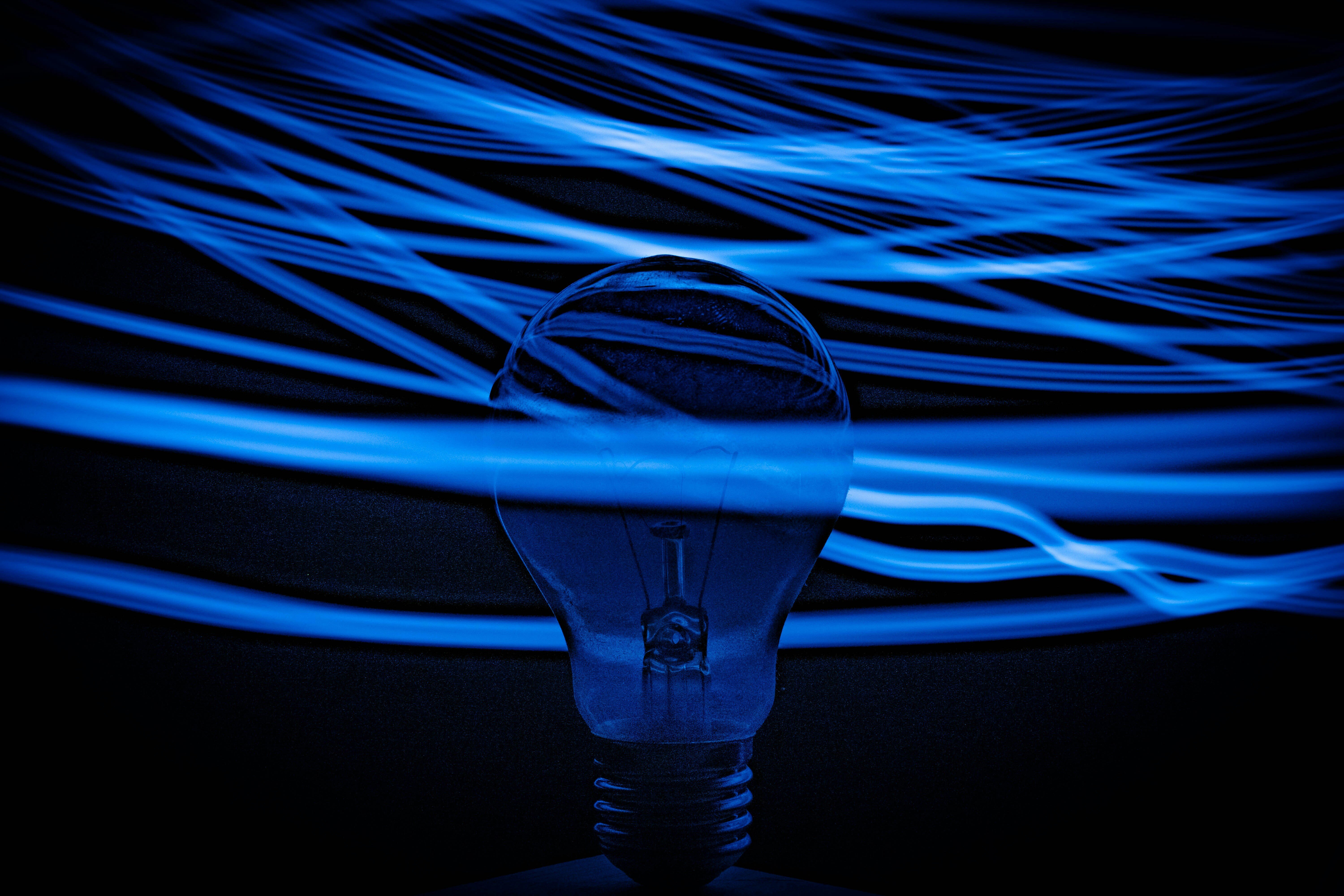
Stravito Included in The Knowledge Management Solutions Landscape, Q3 2024
Charlotte Birchley Sep 11, 2024