Knocking Down the Insights Ivory Tower
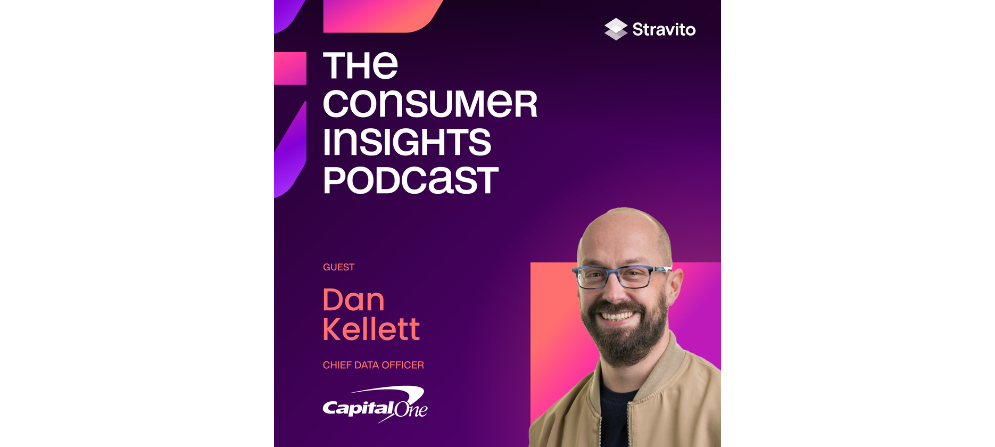
In this episode of the Consumer Insights Podcast, we speak with Dan Kellett, Chief Data Officer at Capital One.
The only thing certain is change – even more so in today’s current macro climate.
And that’s why there’s never been a greater need for data and insights professionals to help business leaders understand how to prepare and react.
But to achieve this, it’s essential to build meaningful connections and to truly dig deep into what matters to stakeholders and customers.
In this episode of the Consumer Insights Podcast, Thor is joined by Dan Kellett, Chief Data Officer at Capital One.
They cover:
- How to take the leap from insight to action
- Why it’s essential to build networks across the organization and avoid an insights ivory tower
- How customer-centric solutions can benefit customers and cut costs
- Why you should never stop at the first answer to the question “Why?”
- The intra-team and inter-team dynamics that create an engaged, inclusive, and impactful data culture
- Why you should never put technology before the business problem
- Why the unglamorous side of data science is crucial
- Building your “techniques bookshelf” to bring the right skills to every problem
- The relationship between data strategy and business strategy
- Why data and insights work today is a team sport
- The importance of celebrating and selling your team's accomplishments
- Navigating the hype cycle
If you’re interested in learning how to knock down the ivory tower of data and insights for more business impact, listen to this episode of The Consumer Insights Podcast.
You can access all episodes of the Consumer Insights Podcast on Apple, Spotify, Google, or use the RSS feed with your favorite player. Below, you'll find a lightly edited transcript of this episode.
Thor Olof Philogène: Hello, everyone, and welcome to The Consumer Insights Podcast. Today, I'm excited to have a brilliant insights leader joining me for what I know will be a fantastic conversation.
I'm thrilled to introduce today's guest, Dan Kellett, Chief Data Officer at Capital One, a company he has worked at for 21 years. Starting as a statistician directly out of school and progressing all the way to his current role. Thank you so much for joining me today, Dan.
Dan Kellett: Great to be here.
Thor: Firstly, could you take a couple of minutes to tell us a bit about yourself, your journey and how you came to work in the role you are in today? How did it all begin?
Dan: Absolutely. My background is in math and statistics. I studied mathematics at the University of Nottingham, graduated in 2000, and really wanted to apply math to real-world problems. I'd had enough of academia, and I wanted to get out there and start using some of the things I'd learned.
I joined Capital One in the UK as a graduate statistician, and I've been there ever since. When I joined Capital One, I had only been in the UK for about three years and was very much in that kind of rapid growth, fast-moving organization. It was a really great place to join as a graduate and start to learn new skills and do different things.
When I initially joined, some of the first things we were doing were things like building and maintaining the models that we used to make predictions on our marketing so to help decide who to send credit card offers to, who are most likely to respond to us, and then who are we most likely to accept.
Building those models and deploying them and then making sure that they continue to work were some of my first jobs. Then over the next five years, I worked on a range of different models and analyses across the whole customer life cycle from marketing through new customer acquisition, onto existing customer management.
Also quite a bit of operational use and even some people analytics as well. I really got to play with data from across the entire customer life cycle.
Then, at that point, I stepped into a small team leadership role, providing statistical support to some test European businesses we had at that time, working with some of our teams at that point in Spain, Italy, and France, which again was a great opportunity to travel and to do some different things.
I took overall leadership for the UK statistical team in 2012 and moved into a Director of Statistics role, really responsible for the overall team strategy, the delivery, the recruitment, and development.
A big part of that at that period was a job family transition to bring in a greater breadth of data science skills and move us beyond just statistical skills. I've grown that team ever since.
Two years ago, I moved into the UK's Chief Data Officer role, and this really encompasses all data job families effectively. We have data analytics, data stewardship, data science, some analytic engineering, and data products.
Really kind of bringing together all aspects of data. This has really helped us benefit from the ability to focus on what really matters and break down silos. Two years into this role and really enjoying it.
How the way Dan works with data has evolved
Thor: Now, you've worked at Capital One for more than 20 years now, which is really impressive. I can imagine that you've changed quite a bit in that time. Could you tell us a bit about how the way you work with data has evolved?
Dan: Yes. I'm often asked, "Why have you been there so long?", when I talk about my story. I think it's really because the role and the company have both evolved as my career has developed.
Every time I've looked to bring in new skills or take my career in a different direction, I've been able to do that from within Capital One. I feel very fortunate that I've been able to grow and develop at the same time as the organization.
As I said before, when I joined, it was very much fast-paced, lots of stuff happening at the same time. Just reflecting on it, there are some really clear differences between then and now.
Two things in particular when it comes to data. Firstly, I feel like we have a much greater emphasis on team delivery now rather than individual delivery. If I think about when I joined, you were almost given your own projects as a statistician, "Okay, you need to look after this model."
Where we are now actually is just that acknowledgment that there are so many different skills, so much different expertise you need to bring together to have successful data science projects, that you can't do it all on your own. It's much more of a team sport. That's definitely one of the big changes.
You would then kind of go away, pull data, build a model, deploy that model. Maybe that would take 9 to 12 months, but that was your project, and so you would do everything around it.
Where we are now actually is just that acknowledgment that there are so many different skills, so much different expertise you need to bring together to have successful data science projects, that you can't do it all on your own. It's much more of a team sport. That's definitely one of the big changes.
The other is the amount that technology has really unlocked processes to move at a much more rapid pace. It's really amazing when I think about some of the models and data pools and analyses that I used to do that would run and take days on end.
One thing that hasn't changed is that nothing stays the same. If I reflect over the 20 years, there's always been something changing, whether that's in the market, whether it's in the wider external environment. I think about the financial crisis, 2007, obviously pandemic response, and now, cost of living.
You would almost set your query going and have to come back to it at the end of the week. Now because of the ability to unlock things like parallel processing and more effective ways of analyzing data, it's much quicker to get to those things. Now, what that means is you've got to be really focused on what you need and more reactive, but I think that's really helped.
One thing that hasn't changed is that nothing stays the same. If I reflect over the 20 years, there's always been something changing, whether that's in the market, whether it's in the wider external environment. I think about the financial crisis, 2007, obviously pandemic response, and now, cost of living.
There's all kinds of things that we just need to be aware of, and actually, that's one of the reasons why it's really exciting being a data professional, is that you're always reacting to new things.
How Dan defines an insight
Thor: I think what you just described is fascinating because a 20-year period is a very long time. As you pointed out, the macro environment has changed many times over, and so has the technological evolution. I thought it was fascinating, even the way teams work.
On your LinkedIn, you write that, "Making sense of the world by turning data to insight to action." Can you tell us a bit about what your journey looks like? Talking about this, how do you also define an insight?
Dan: For me, insight is something that helps people understand the “why?” for the reasons of helping the “what next?”. Now, it's relatively easy to collect a lot of data, I don't think that is getting your job done.
You can instrument everything, you can bring everything into a data lake or however you want to store your data, but really, if you just do that, you run the risk of just adding more hay and not adding any more needles.
That for me is not insights. That for me is data collection, which is really important, you need to have the data, you need to trust it, it needs to be good quality, but that's not going to get you insights and to action.
You can instrument everything, you can bring everything into a data lake or however you want to store your data, but really, if you just do that, you run the risk of just adding more hay and not adding any more needles.
The first part of that then is understanding the “why”. For me, that's a big part of really understanding what makes the organization that you're working for tick. “How do you better understand your customer needs? How do you better understand your stakeholders and what matters to them, keeps them awake at night?”
The more you understand that, the better you're able to target the fact that you have all this data in a way that really helps you understand and gather, "Ah, I can find this, and that might help answer this question." That helps you with the “why”, but I think where it really becomes super valuable is the “what next”.
It's great if you can find a trend or a pattern and you can say, "Hey, this group of customers are acting in this particular way," but unless you can then take it to the next step and go, “and as such, I think we ought to change our strategy”, or “I think we ought to offer this different product to this group”, that's really where the gold is. That's what unlocks the power of the data for me.
Taking the leap from insight to action
Thor: I really like that. I think in terms of maybe educating our audience and helping the audience benefit from your experiences, could you tell us a bit more about that final leap from insight to action? Do you have any examples you could share?
Dan: Absolutely. For Capital One, as a credit provider, one of the most important decisions that we make is whether to lend or not. Our customer applies to us and says, "I would like a Capital One credit card."
We've got a big decision to make. Do we say yes or no? Now, we need to make sure that decision is right for us and right for the customer. Data and the models that we build within the team provide the bedrock, the foundation of those decisions.
We build machine learning models that look at historical data and try to predict whether it would be the right decision to offer that credit card to the customer or not.
Now we’ve had these models in place for decades now, but how do you improve these decisions? That’s what in part we continue to focus on. How do you make sure that these decisions continue to get better and better? In my mind there are three ways that you can do that.
You can make sure that your data is up to date as possible, so that it reflects the freshest insights, the freshest signals you can find. You could bring in new data sources, different ways of thinking about the customer, and you can also use new techniques, so you can push the development of which machine learning techniques you use.
All three of those, in my experience, really help in terms of pushing the boundaries and making sure your model continues to be as powerful as possible.
As a team, we're always looking for those opportunities within those three areas but just finding those opportunities isn't enough, until those opportunities have been baked into a new model that's then been deployed and is actually making decisions in the real world, I don't think our job is done.
That's one example, I guess, where we're always looking for things out there, but it has to be baked into that wider process to make sure that we're actually using that data to actually make better decisions.
Using insights to fuel innovation
Thor: Then I think it's really interesting to see how much at the core of the business your team is, because, as you said, that credit decision is one of the most important things Capital One does, right?
You're very at the heart of the business. Maybe we talk a bit more about your time at Capital One and maybe you could give us an example of a time when your team has integrated insights that have fueled innovation and perhaps allowed you to build a better product, a better campaign, or a better project.
Dan: Yes, definitely. If I think about an example from a few years ago, now we have a tech team and a product team within Capital One who look after our mobile app, making sure that the app that we build and maintain offers the best customer experience.
Now, you wouldn't be surprised here. We have a long backlog of features. There's always more things you could build into that app, and one of the questions that we were asked is “How do we prioritize and really understand the impact of some of these potential future features so that we can actually pick the features that have the most customer impact?”
Now, one of the things that you want your mobile app to do is to make it really easy for customers to get whatever they want done. Actually, if they can't do that in the app and they probably call you. Now, when a customer calls us, it's less convenient for the customer. Also, it's more costly for us.
Ideally, we want customers to be able to do whatever they need to within the app. We decided to go and look at some of the records that our call center associates took as part of calls over the previous six months. These are really interesting notes. There's lots of detail in there. It's very unstructured data. There's lots of acronyms and all kinds of stuff in there.
The team built up that feature, and we actually saw a big switch away from calls coming in to make payments and more people making payments in the app.
That's an example of where your data insights doesn't have to be a deployed model or a decision-making model. Actually, a one-off piece of analysis can provide some real insight that helps drive forward the overall business strategy.
There's a real challenge to clean that data and really understand it, but actually it's a gold mine for information. The team was able to take some of those notes, mine it, using some natural language processing techniques and cluster the notes up.
Now, what that allowed us to do, is to go, "There's a whole load of calls here that are about this subject, a whole load of calls over here about this subject. Let's pick the biggest one," and what we were finding was a similar thread within all the calls in that biggest cluster were around payments and “How can I make a payment more easily?”
We were able to take that insight back to the mobile app team and say, "Hey, you've got a load of calls coming in here. Actually, if you build some functionality to improve that payment journey into the app, we think that the customers will just use the app to do that and not call us."
The team built up that feature, and we actually saw a big switch away from calls coming in to make payments and more people making payments in the app.
That's an example of where your data insights doesn't have to be a deployed model or a decision-making model. Actually, a one-off piece of analysis can provide some real insight that helps drive forward the overall business strategy.
Identifying insights
Thor: I think that's a fascinating example, and I think it really emphasizes the importance of that tight connection between teams. You make it sound so easy. Any advice for any of our listeners on how you actually identified those insights? Because the clustering sounds so logical. Tell us a bit more about that.
Dan: Yes. I don't think it is logical. I think it is all around really digging to understand what's important. One of the things I often say to new members in the team is “keep asking why, don't stop at the first answer” as well.
If someone tells you this is important, ask them why it's important, and then whatever they tell you, ask them again, because what you find is it the first answer may well not be the root of the reason why this is important.
The more you question, the more you ask, the better you're going to understand what really matters, and the better you can focus your effort and time onto things that will solve that. I think there's a little bit of trial and error, but for me, it is all around networks and building those links with different people across the organization.
What the right data culture looks like
Thor: I absolutely love that advice because what you're telling us is really to-- you're really giving us advice in terms of how to think.
You've got a great microblog on LinkedIn. One of the themes that come up frequently in your posts is the importance of creating the right culture, where insights are integrated into organizational processes. Could you tell us a bit more about what the right data culture looks like and how to achieve it?
Dan: Yes. I think this was something I spent a lot of time thinking about moving into the Chief Data Officer role.
Obviously, we have all these different data disciplines, how do you make sure you're building a really engaged, inclusive culture that is able to have a really effective impact on the business? I think there's things that you can do within the team, and there's things that you can do with the way that the team looks externally.
Within the team, it's really important to have a high degree of transparency, a lot of openness as to what's happening within the team so people can get involved, put their hand up and say, "Hey, I like the look of what's going on over there. How do I get closer to that?" I think that that level of transparency is important.
I'm really wary that we don't want to build some kind of ivory tower where people go, "Oh, that's the data team over there. You have to book three months in advance if you ever want to speak to anybody over there."
We make sure we have a really strong culture of recognition. We have some amazing individuals in the team, and it's really exciting to be able to recognize when you see some really strong results coming out from the team, or even just well-thought-through work. It doesn't always necessarily have to translate into results. I think we do a good job of recognizing just great data professionalism.
I think the other thing is a clarity of vision. “Why do we exist as a team?” Having a stake in the ground and going "This is what we stand for, and this is how we organize ourselves.", helps people.
And then finally, just have some fun. We spend a lot of our time at work. It should be an organization that's exciting and fun and interesting. That's another thing that we need to think about. They're all the things of how do you build that culture internally?
The external-facing stuff I think is also important. I'm really wary that we don't want to build some kind of ivory tower where people go, "Oh, that's the data team over there. You have to book three months in advance if you ever want to speak to anybody over there."
If that's the way that we're perceived, then it's not working. We need to make sure that we obsess around building those networks and that we're always focused on delivering the things that matter most.
What not to do as an insights leader
Thor: Let's avoid the ivory tower, clear. I also really like the idea of data karma. Maybe there are any other things that you'd like to highlight that we should avoid doing?
Dan: When I've seen it all go wrong, I think sometimes when timelines get tight or things don't go to plan, that's when it can all get a bit stressful. I think if you don't have the goodwill in the bank, people are more likely to draw their own conclusions or go off and do things the other way.
The data karma aspect is brilliant. I completely agree with you there. It's all about how you build networks in a good time so that you can rely on those things when you really need to. I think the more time you invest into that, the better. Actually, if you avoid doing that, that's when it kind of all falls apart.
Aligning data projects with real business problems
Thor: I absolutely love that. One of your many accomplishments has been the creation and development of the UK data labs, including the application of big data analytics to real-world business problems.
From your perspective, what are some of the best strategies to ensure that data projects stay aligned with the real business problems that they're trying to solve and how do you balance the long-term business needs like foresight, with the short-term business needs like the agility in the face of rapid consumer shifts or other changes?
Dan: This is an area where I feel like I've learned a lot through it working and it not working over the time. There have been periods of my career where it has potentially fallen into that ivory tower situation, where you've got some new technology that you're looking into and you tend to kind of go away.
The risk sometimes is you put the technology first before the business problem, and so you go, "Hey, we must learn about X," and you learn about X, whatever X is, and you go, "Right, how can we apply X to the problems we have?" That's where, in my career, it's been much harder to get that buy-in.
It's been much easier to work first on one of the business problems and then see how we can apply some of the new technologies or approaches. An analogy I always tend to use is I think a great data scientist almost has a bookshelf of techniques, and they know exactly when to reach for which book. Arithmetic means sometimes the best result, the best thing you can use.
As a great data scientist, you should always be building your professional knowledge and capabilities so that you're keeping that bookshelf stacked but then you also need to reach for the right book at the right time.
Keeping business stakeholders engaged
Thor: Yes, I think the bookshelf analogy is very clear and helpful for us as we can relate to it, having been trained in different elements and different areas. In order to support successful data and insight strategy, which tools do you believe are essential? In particular, how do you ensure that broader business stakeholders are able to stay engaged?
Dan: Yes. I think in terms of tools and technologies in particular, I'm reasonably agnostic to it. It's more around what the capabilities are that need to be in place for a successful data and insight strategy.
Firstly, I think you need to be able to trust your data. Potentially, this is an area that gets overlooked for the more glamorous data science side of things, but actually, if you build your models and your insights on rubbish data, you're going to get rubbish models and rubbish insights.
You really need to trust that data, which is why our data stewardship team is such an important team to us. Being able to access, trust, use, understand that data I think is the first part of that.
You really need to trust that data, which is why our data stewardship team is such an important team to us. Being able to access, trust, use, understand that data I think is the first part of that.
The second thing is the skills that you need to analyze and model your data. No data comes beautifully prepared. I think as a data scientist, you've got to be good at doing that data manipulation, that data understanding, data cleaning.
Probably 70% of the time is spent doing that. Again, it's not the glamorous stuff, but it's the stuff that actually underpins the success. There's all that data cleaning stuff. Then you can get to do your algorithmic work, build your models, do your analysis, pull out those insights.
Then the final bit is how you communicate and visualize those results. Again, I think that has to be a core skill within a data scientist's locker, the ability to not just talk models but to talk about the "so what's" and to be able to pitch your analysis and results to different audiences and to be able to explain what that means. That's when you really get successful. That's when it's really important.
Then the final bit is how you communicate and visualize those results. Again, I think that has to be a core skill within a data scientist's locker, the ability to not just talk models but to talk about the "so what's" and to be able to pitch your analysis and results to different audiences and to be able to explain what that means.
You asked, "How do you keep your stakeholders engaged through that process?" Well, I think it's really important that whatever your data strategy is, that it's linked to your business strategy. They can't be two divergent strategies. I think the business strategy has to come first and then it's underpinned by a really strong data strategy.
Now, a key part of my role as a Chief Data Officer for the UK business is really to keep on top of that stakeholder engagement, to make sure there's regular communications, that we're celebrating successes, and to really kind of build that team identity.
Also, a bit of a sales mentality, which doesn't naturally always come to data professionals to the extent where I don't like to talk about it as being sales. This is about talking about what you've done.
If you've built a model or get some insight that you think is really powerful and insightful, if you're not selling that or talking about it or shouting about it, then it goes nowhere. That has to be a key part of how you continue to work with business stakeholders.
Essential skills to elevate data and insights within the organization
Thor: It's so relevant, and it's also tying nicely back to what you said before, which is, never create a situation where technology comes first before the business problem.
If we talk a bit and if we dig a little bit deeper into this and we talk a bit about the skills, you've talked about the sales mentality, which is really more about being able to talk about and be proud of the work you've done. What skills do you believe to be essential to help elevate data and insights within an organization?
Dan: I think there's a whole range of technology skills that are really important. Now, do I think an individual has to have all those skills? Not at all. Everybody has seen those pictures on LinkedIn of every skill that a data scientist needs. That's just not realistic.
What I think you need is those skills within the team. We have a really strong concept of “If you bring your skills and experience to the team, and actually as a team, you end up having deep experience and skills across a wide variety of different areas that help you succeed.”
What that means is not only you need to make sure that you're continuing to look at the overall makeup of your team in terms of technology skills, it also means people need to be able to work in a team.
We can teach technology skills, we can teach people to code, we can teach people how to build models and validate models. What I think is much harder to teach is that innate curiosity to ask questions. I think that it really is a superpower.
This is not a game for individuals in my mind. I think it's something where you need to have a really strong ability to work with other people, strong communication skills, and really all focus on a single goal.
The other thing that I think is really important is curiosity. Again, when I'm talking to university students or people who are looking to join our team, that's the one thing I'm looking for, is I'm looking for that curiosity to really ask those questions why and understand what's happening.
We can teach technology skills, we can teach people to code, we can teach people how to build models and validate models. What I think is much harder to teach is that innate curiosity to ask questions. I think that it really is a superpower.
Opportunities for data and insights professionals to challenge the status quo
Thor: I really like what you just said. I think it ties very nicely to what you said in the very beginning of our conversation where you said that one big evolution is that more skills need to be brought together, it's more of a team sport today than it used to be.
Looking ahead, what opportunities do you think there are for data and insights professionals? How can they make a true business impact? How can they challenge the status quo?
Dan: We talked about the fact that things are always changing, but actually at the moment, it's a real time of extreme change in pretty much every dimension you want to talk about.
What that means is that there's never been a greater need for data professionals to try and help business leaders understand what's happening. I just see there is so much appetite out there from business leaders to say, "We've been collecting this data. How do we understand it? How do we make sense of this rapidly changing world that we're in?"
There is the opportunity to be able to walk in the door and take the data that's been collected and turn it into insight, turn it into future strategy, and help answer some of those questions that are actually leading to a lot of senior executives to wonder what's next. I think data really helps with that. For me, that's the opportunity.
Challenges on the horizon for data and insights professionals
Thor: On the flip side, because I think your point is super crisp and clear and will resonate with many of us, what challenges do you see on the other side? What is it that could face data and insights professionals and the wider industry in the near future?
Dan: It's the hype cycle. On one hand, I feel really fortunate to have developed my career into an area that is really hot at the moment. There's a lot of attention. There's a lot of money being pumped towards data strategy and machine learning, and so on.
My worry is that it gets oversold and the expectations become way beyond what can actually be delivered. It's a really delicate balance, because I don't want to be going, "Oh, no, we can't do that," but actually, equally, I want to make sure that we're not over-promising. I think that's really important, is to keep on top of that hype.
Who in the world of insights Dan would love to have lunch with
Thor: Dan, I really appreciate that you're grounding this conversation. I think you're bringing some very important elements to reality. Things can get caught up in the hype cycle, definitely.
Unfortunately, we're getting close to the end of this conversation, which really hurts me, but I would really like for you as a last question to tell me who in the world of data and insights would you love to have lunch with?
Dan: Oh, that is such a difficult question. I love finding out about what data professionals are doing in other industries. I think it's really important that data professionals aren't just focused within, whether that's within organization or within their industry.
I get my ideas, I get my “What next?” from talking to people in other industries. Now, by nature, I'm actually an introvert, and the idea of having lunch with someone I don't know used to fill me with absolute dread.
Actually, if you're able to frame that around, "What are you doing? Tell me about that. Tell me about what data you use. Tell me what techniques you're using. How do you generate your culture?" That's the area that gets me really interested.
I guess to answer your question, anyone from different industries who's really pushing the boundaries of data and insight, if you're out there and you're listening and you fancy a chat, get in touch.
Summary
Thor: I love that. I think it forces you to think differently about the problems you're solving. I understand why that makes perfect sense. Wow, this has been such an interesting conversation, Dan.
It's been amazing to hear about how you and Capital One are leveraging data to fuel more insights-driven decisions across the organization. I love how you reminded us that data and insights are now forcing people to bring more skills together. It's less of an individual sport, it's much more of a team sport. We need to avoid the ivory tower together.
We should never put technology first and never before the actual business problems we're looking to solve. The ability to not just talk models, when you seek out to build out your teams, need to be core of any data scientist. Frankly speaking, people need to have a bit of a sales mentality.
Lastly, Dan, as a Chief Data Officer, I never thought you would tell me not to put technology first, and also hire somebody who has curiosity because you can teach the technology skills. I just love it. I know that I've learned a lot from talking to you today, and I'm sure our audience has as well. Thank you so much for joining me today.
Dan: Thanks. It's been a pleasure.
Related Content
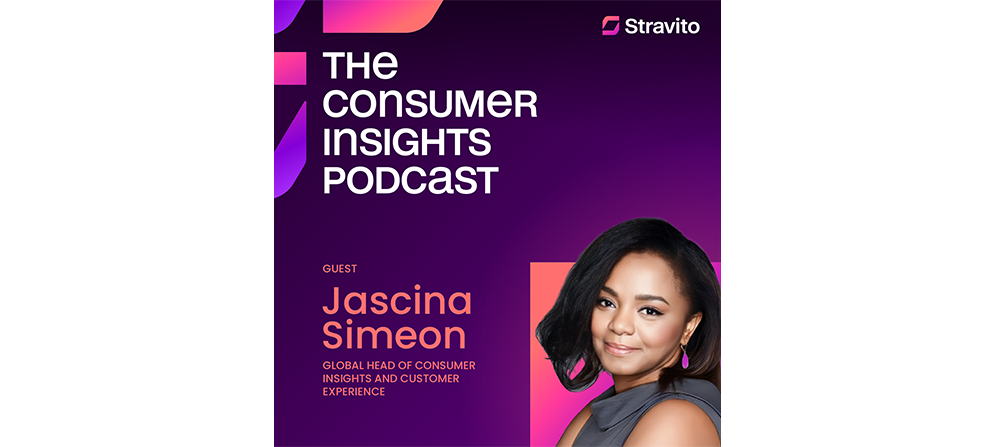
The Power of Asking the Right Questions
Stravito Jun 20, 2024
In this episode of the Consumer Insights Podcast, Thor is joined by Jascina Simeon, Global Head of Consumer Insights and Customer Experience.
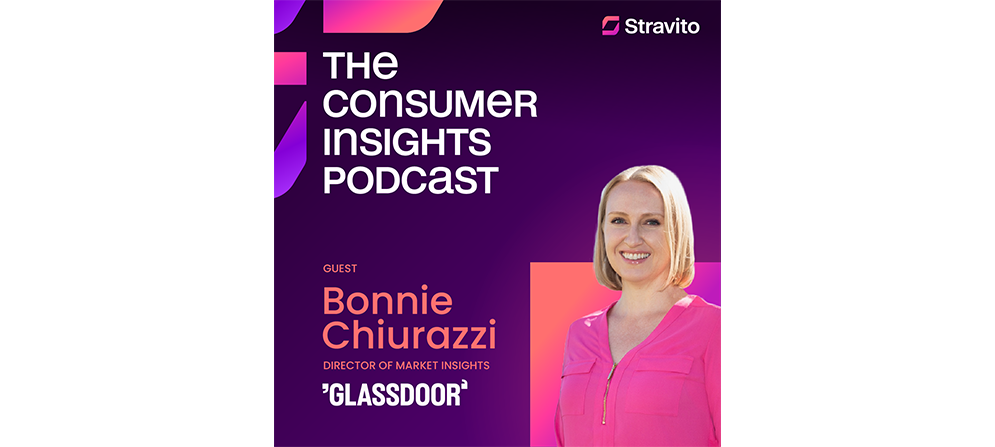
Real-World Insights
Stravito Jun 6, 2024
In this episode of the Consumer Insights Podcast, Thor is joined by Bonnie Chiurazzi, Director of Market Insights at Glassdoor.
Subscribe to the latest podcast updates
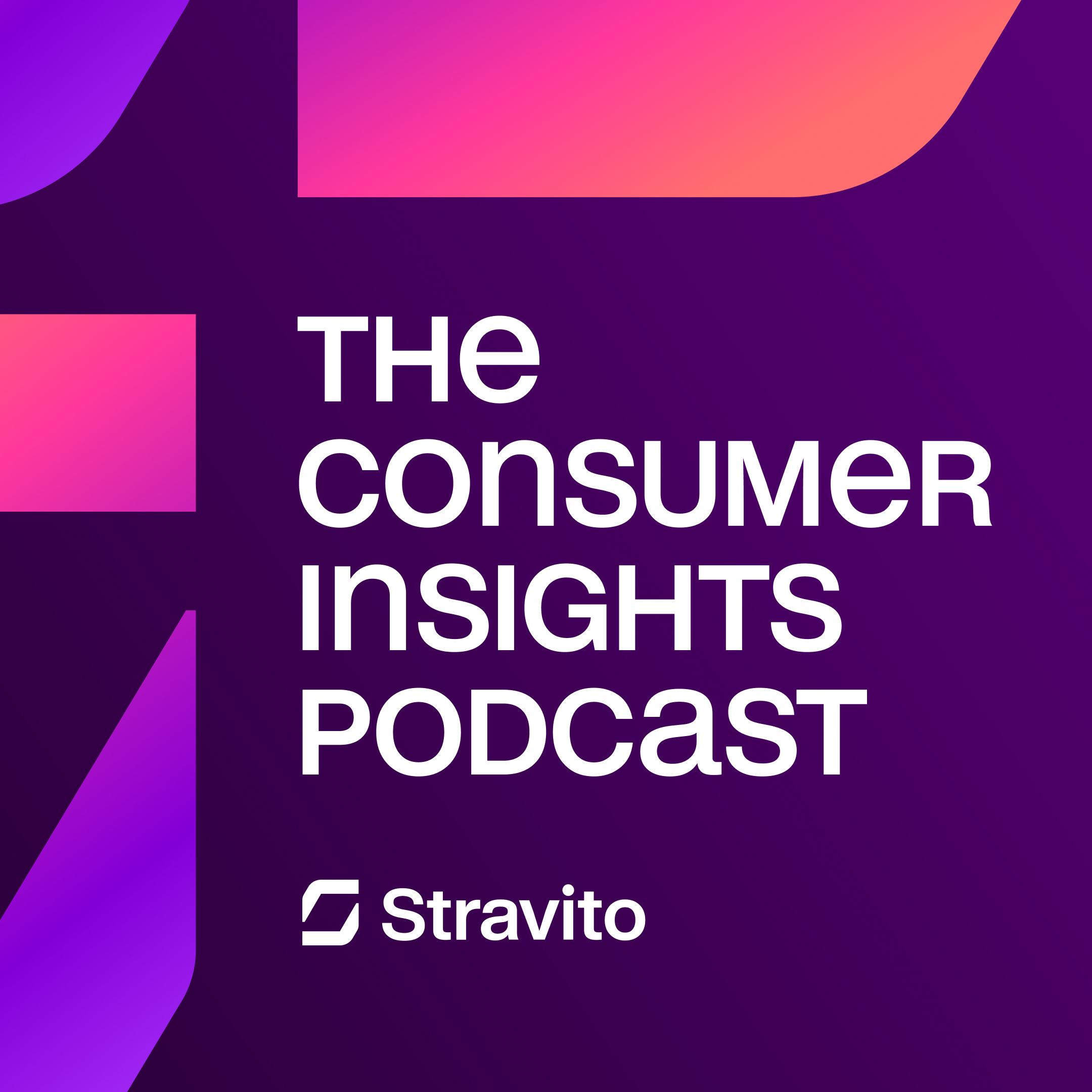